Method
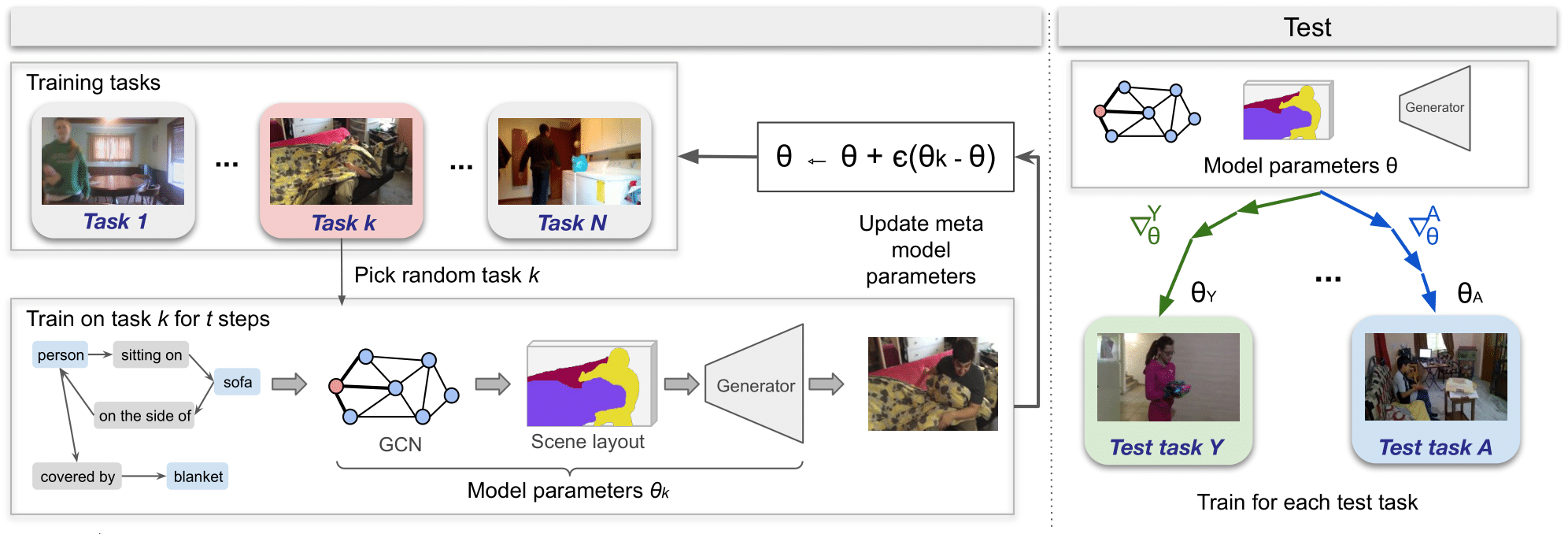
Abstract
Generation of images from scene graphs is a promising direction towards explicit scene generation and manipulation. However, the images generated from the scene graphs lack quality, which in part comes due to high difficulty and diversity in the data. We propose MIGS (Meta Image Generation from Scene Graphs), a meta-learning based approach for few-shot image generation from graphs that enables adapting the model to different scenes and increases the image quality by training on diverse sets of tasks. By sampling the data in a task-driven fashion, we train the generator using meta-learning on different sets of tasks that are categorized based on the scene attributes. Our results show that using this meta-learning approach for the generation of images from scene graphs achieves state-of-the-art performance in terms of image quality and capturing the semantic relationships in the scene.
Contributions
- A novel meta-learning approach for the generation of images from scene graphs, which achieves state-of-the-art results compared to previous work
- Introduction of the few-shot learning problem for scene graph to image generation
- A novel task sampling method for scenes in the wild that can benefit other image generation with meta-learning scenarios.
Paper
British Machine Vision Conference (BMVC) 2021Paper PDF | arXiv
@inproceedings{farshad2021_MIGS, title={MIGS: Meta Image Generation from Scene Graphs}, author={Farshad, Azade and Musatian, Sabrina and Dhamo, Helisa and Navab, Nassir}, booktitle={BMVC}, year={2021} }
Source Code & Checkpoints
Downloads
The pretrained model checkpoint are available at: CheckpointsSource Code
The source code is publicly available in the following repository: Source CodeTeam
Our Team
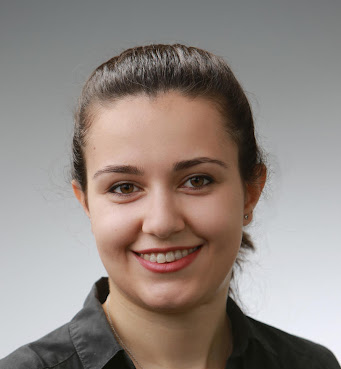
Azade Farshad
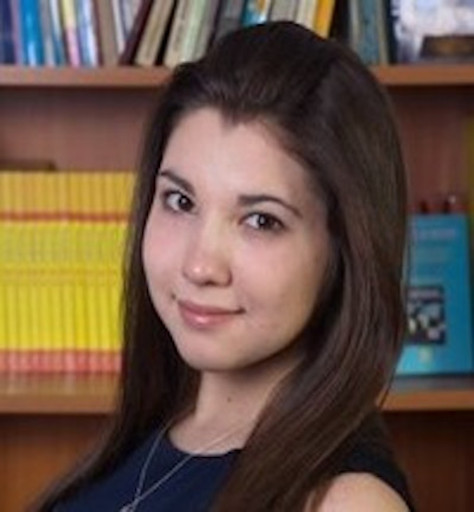
Sabrina Musatian
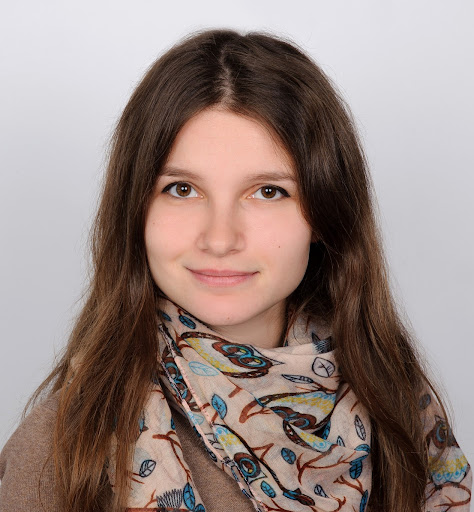
Helisa Dhamo
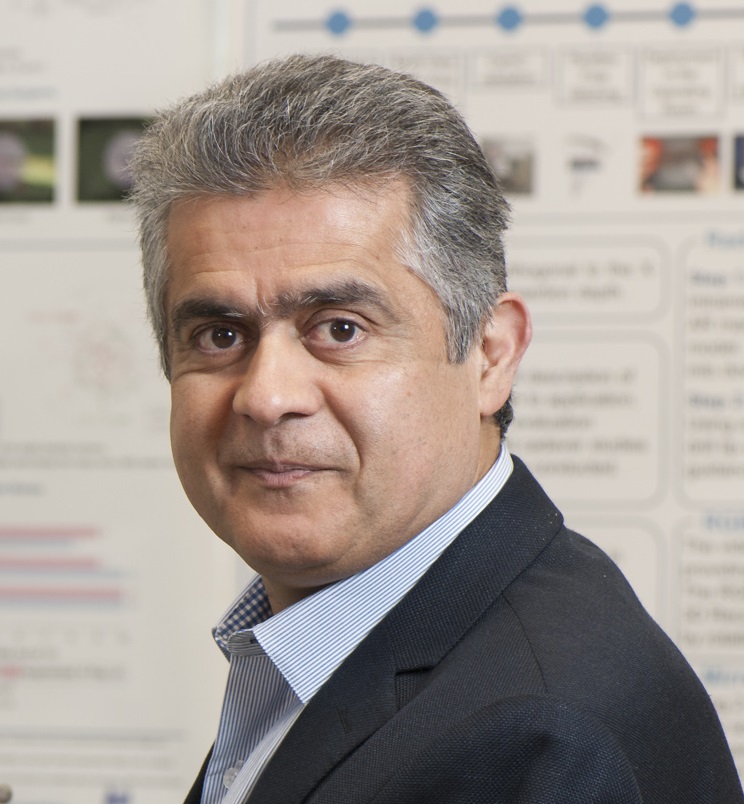
Nassir Navab
Contact
Contact Us
In case you have any questions or you are looking for collaborations, feel free to contact us.
Email:
azade.farshad@tum.de
sabrina.musatian@tum.de